Document Type
Article
Publication Date
1-2009
Publication Title
Neural Computing and Applications
Volume
18
Issue
1
Pages
37-44
Abstract
Monthly Federal Fund interest rate values, set by the Federal Open Market Committee, have been the subject of much speculation prior to the announcement of their new values each period. In this study we use four competing methodologies to model and forecast the behavior of these short term Federal Fund interest rates. These methodologies are: time series, Taylor, econometric and neural network. The time series forecasts use only past values of Federal Funds rates. The celebrated Taylor rule methodology theorizes that the Federal Fund rate values are influenced solely by deviations from a desired level of inflation and from potential output. The econometric and neural network models have inputs used by both the time series and Taylor rule. Using monthly data from 1958 to the end of 2005 we distinguish between sample and out-of-sample sets to train, evaluate, and compare the models’ effectiveness. Our results indicate that the econometric modeling performs better than the other approaches when the data are divided into two sets of pre-Greenspan and Greenspan periods. However, when the data sample is divided into three groups of low, medium and high Federal Funds, the neural network approach does best.
Recommended Citation
Malliaris, Anastasios G. and Malliaris, Mary. Modeling Federal Funds Rates: A Comparison of Four Methodologies. Neural Computing and Applications, 18, 1: 37-44, 2009. Retrieved from Loyola eCommons, School of Business: Faculty Publications and Other Works, http://dx.doi.org/10.1007/s00521-008-0198-y
Creative Commons License
This work is licensed under a Creative Commons Attribution-Noncommercial-No Derivative Works 3.0 License.
Copyright Statement
© Springer International Publishing AG 2009
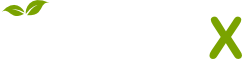
- Citations
- Citation Indexes: 2
- Policy Citations: 2
- Usage
- Downloads: 1444
- Abstract Views: 9
- Captures
- Readers: 8
Comments
Author Posting. © Springer International Publishing AG 2009. This is the authors' version of the work. It is posted here by permission of Neural Computing and Applications for personal use, not for redistribution. the definitive version was published in Neural Computing and Applications, vol. 18, no. 1, 2009, https://link.springer.com/article/10.1007/s00521-008-0198-y